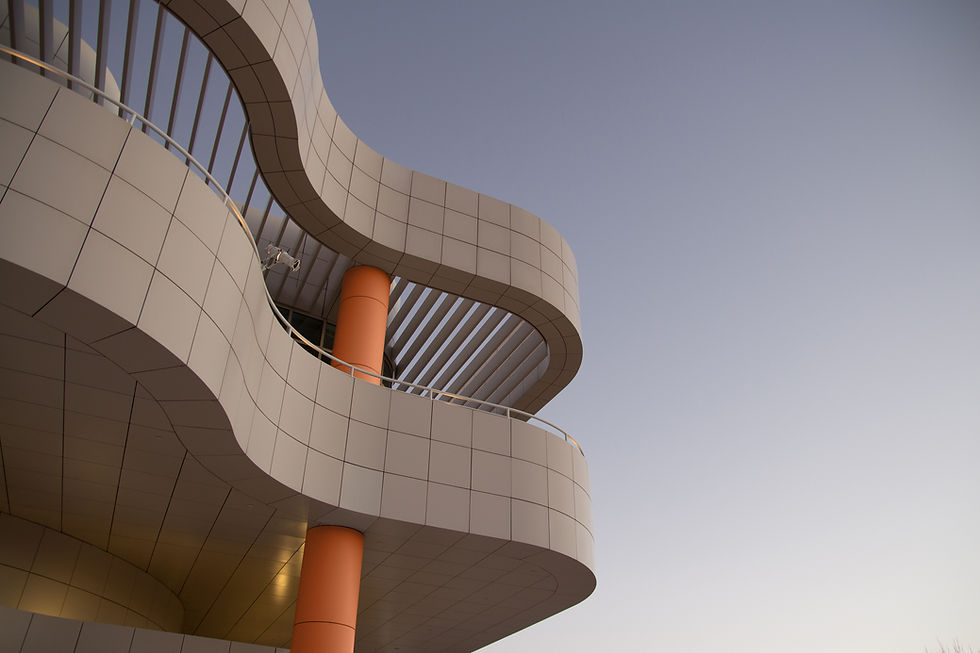
Microsoft's Large Action Models: Redefining AI with Real-World Task Execution
Jan 14
3 min read
0
0
0
Microsoft has unveiled a groundbreaking advancement in artificial intelligence by introducing Large Action Models (LAMs). These AI systems mark a significant leap from traditional large language models (LLMs) by processing and generating text and performing complex tasks in real-world environments. Designed to operate Windows programs autonomously, LAMs represent a pivotal shift in AI development, moving from systems that discuss functions to those that can execute them.
Large Action Models go beyond the capabilities of LLMs, which excel at understanding and generating text but face limitations in translating user inputs into actionable steps. Unlike their predecessors, LAMs can perform tasks such as operating software or controlling devices. For instance, while traditional models like GPT-4o can explain how to shop online, LAMs can navigate an interface and complete the shopping process independently. This revolutionary capability extends to Microsoft Office applications, where LAMs can execute tasks such as creating, formatting, and organizing presentations or documents based on human instructions.

LAMs are trained to process diverse inputs, including text, voice, or images, and convert them into detailed action plans. They are also capable of dynamically adapting their actions based on real-time feedback. This adaptability ensures that LAMs can handle tasks even in evolving scenarios, making them a practical tool for digital and physical environments.
Building a LAM is a complex process involving multiple stages of development. The training begins with teaching the model to break tasks into logical steps. Subsequently, the model learns to translate these plans into actions, often leveraging advanced AI systems like GPT-4o as a foundation. It then autonomously explores innovative solutions, tackling problems other AI systems may struggle with. The final phase involves fine-tuning through reward-based training to optimize performance. The research team tested a LAM built on the Mistral-7B model within a Word test environment, where it completed tasks 71% of the time—significantly outperforming GPT-4o's 63% success rate without visual inputs. Additionally, LAMs demonstrated superior efficiency, completing tasks in 30 seconds compared to GPT-4o's 86 seconds.
The training process for LAMs also involves the creation of extensive datasets. The Microsoft team began with 29,000 task-plan pairs sourced from documentation, wikiHow articles, and Bing searches. This dataset was expanded using a "data evolving" strategy, where simple tasks were transformed into more complex scenarios. For example, a task like "Create a drop-down list" was enhanced into "Create a dependent drop-down list where the first selection filters the options in the second list." This approach expanded the dataset by 150%, resulting in 76,000 pairs, with 2,000 successful action sequences forming the core training set.

Despite these advancements, the journey to fully scalable LAMs is challenging. Concerns about potential errors in AI actions, regulatory issues, and technical hurdles remain. Nevertheless, the researchers believe LAMs represent a transformative step toward artificial general intelligence (AGI). Unlike traditional AI models that are limited to interpreting and generating text, LAMs have the potential to actively assist in completing real-world tasks, heralding a future where AI systems seamlessly integrate into everyday workflows.
As the technology matures, the implications of LAMs extend across industries. From automating workflows to supporting individuals with disabilities, the applications are vast and varied. By bridging the gap between understanding and action, Microsoft's Large Action Models are set to redefine the capabilities of AI, offering a glimpse into a future where machines not only comprehend our needs but also act upon them with precision and adaptability.