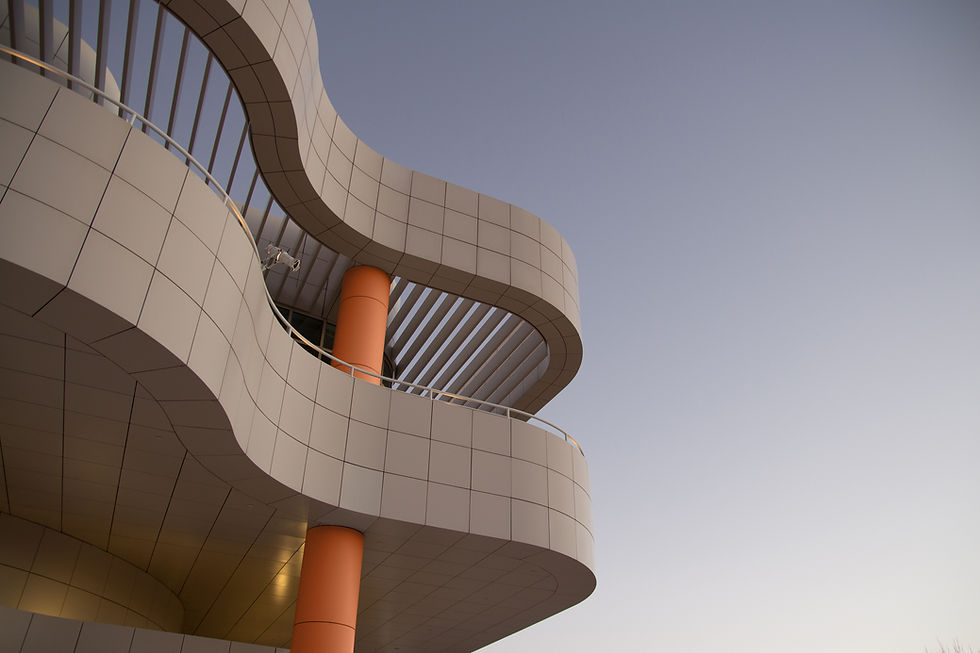
AI Surpasses Human Experts in Predicting Neuroscience Study Outcomes
Nov 27, 2024
3 min read
0
2
0
A groundbreaking study led by researchers at University College London (UCL) has revealed that artificial intelligence (AI) can predict the results of neuroscience studies with remarkable accuracy, surpassing the capabilities of human experts. Published in Nature Human Behaviour, the study demonstrates how large language models (LLMs), a type of AI designed to analyze vast text datasets, can identify patterns in scientific literature and forecast experimental outcomes with superhuman precision. This milestone in AI research highlights the transformative potential of these technologies in accelerating scientific discovery and innovation.
The research team, led by Dr. Ken Luo from UCL’s Psychology & Language Sciences department, aimed to explore the predictive capabilities of AI beyond simple knowledge retrieval. Dr. Luo explained that while generative AI systems like ChatGPT are often praised for their ability to summarize and answer questions based on training data, this study focused on whether AI could synthesize existing knowledge to predict future results. The trial-and-error process often hinders scientific progress, which requires extensive time and resources. Even skilled researchers may overlook valuable insights buried in the vast and growing body of scientific literature.

To address this, the team developed BrainBench, an innovative tool designed to evaluate how well large language models can predict neuroscience study results. BrainBench pairs abstracts of neuroscience studies, where one is the original with accurate results, and the other is a version modified by domain experts to include plausible but incorrect outcomes. Both human neuroscience experts and AI models were tasked with identifying the factual study abstract.
The study involved 15 general-purpose AI models and 171 human neuroscience experts who had passed a screening test confirming their expertise. The findings were striking: all AI models outperformed the human experts. The AI models achieved an average accuracy of 81%, while human participants managed only 63%. Even when the analysis was restricted to the most experienced human participants, their accuracy only improved to 66%. The AI’s performance reflects its ability to discern patterns across extensive datasets, a task that often overwhelms human cognition.

To push the boundaries further, the team customized an open-source LLM called Mistral, fine-tuning it on neuroscience literature to create BrainGPT. This specialized model achieved an impressive 86% accuracy, surpassing even the general-purpose Mistral model, which reached 83%. The researchers noted that when AI models expressed higher confidence in their predictions, their accuracy tended to be even more significant, suggesting that AI could become a valuable partner in scientific research when calibrated effectively.
Professor Bradley Love, a senior author from UCL, reflected on the implications of these findings, suggesting they reveal a more profound truth about the nature of scientific research. He observed that many scientific results are only partially novel but conform to existing patterns within the literature. This raises questions about whether researchers must be sufficiently innovative and exploratory in their studies. Dr. Luo echoed these sentiments, envisioning a future where AI tools assist researchers in designing experiments by predicting the likelihood of various outcomes. By inputting their proposed designs and anticipated findings into an AI system, scientists could iterate more rapidly and make more informed decisions.

The study was a collaborative effort involving researchers from institutions worldwide, including the University of Cambridge, the University of Oxford, the Max Planck Institute for Neurobiology of Behavior, and other universities in countries such as the UK, the US, Germany, and Australia. This research not only underscores the power of AI in predicting scientific outcomes but also highlights its potential to transform the way experiments are designed and conducted, paving the way for faster and more efficient scientific advancements.